Building Voice Tech for Education with Mauro Nicolao at Soapbox Labs
Hi and welcome to Fireside AI.
My name is Catherine Breslin and I'm here to talk about how companies build AI technology.
Stay pleased to have Mauro Nicolao from Soapbox Labs here.
Mauro, welcome to the show.
Thank you very much, Catherine, for having me.
I'm very glad to talk with you about all the challenges in AI.
Fantastic.
It's great to have you here today.
We have obviously known each other for some years.
We've worked sort of side by side in the voice technology industry over the years and kept
up with each other that way.
I know that you have sort of started your career at Soapbox Labs.
Tell us a little bit about yourself and how you ended up at Soapbox Labs.
Absolutely.
So at the moment I'm director of data scientists at Soapbox Lab and Soapbox Lab is now a
curriculum associate company.
I started to work with Soapbox Labs like three and a half years ago.
Before that I worked for academia for a long time and I worked in Italy where I'm from and
for a few years and then I moved to Sheffield, University of Sheffield in the UK.
for 10 plus years doing my PhD and my research associate work experience.
I've always been working, as you said, in the speech technologies, basically being in the
university where you're exposed to different projects.
from text to speech, and speech to text, and speaker identification, all sorts of the
aspects of speech technology.
But for some reason I've been always
drawn towards educational projects like EdTech both in Italy and the UK, I ended up being
involved in educational projects.
So it was a natural, let's say, uh evolution for me to move to a company such as Soapbox
Labs where they've been developing children specific voice technology for the last 11
years.
uh
Softbox Labs is a startup built in Dublin.
The team members are super dynamic and exciting people.
They were super proud of the work they were doing.
And so it was, again, natural for me to join them and be starting creating new exciting
features for children.
So because we were quite good at what we were doing, a year and a half ago, we were
acquired by this US publisher.
called Curriculum Associates.
And the acquisition went, I think, extremely well because there were a lot of alignments
in terms of ideas, goals, and visions, and especially in putting children first.
And since then, we merged the speech technology in their product, or in our product.
So I know from experience that children's speech is hard for technology to recognise, but
we'll maybe get onto the technical challenges of that later.
But first, I'm interested to learn a little bit more about what it was like for you to go
from a startup building voice technology and then being acquired by a bigger company.
What was that journey like?
Yeah, as I saying before, transition between merging with CEA was super easy from many
points of view because there was no change in terms of vision, goals and perspective.
Challenges are maybe mostly on the organization side.
So where you have now to deal not just with your 10 colleagues, you now have 10, 1000
colleagues to work with.
I can say so far it's been frantic because I had to learn different processes and ways to
operate in a bigger company.
Also very exciting because we are really getting in front of children.
What I mean by that is before this, were mostly, when we were a startup, we were a backend
service company.
So we were providing voice technology for other publishers.
and they were creating the front end and everything.
Now we are driving the front end creation.
Even we have a say into the development of new metrics or a new methodologies, teaching
methodologies as well.
So I guess one of the challenges of using voice AI in educational space is it changes
everything.
Most of the methodologies were based on limited teacher time.
Using voice AI technologies, unlock a lot of potential and data points and measurements
that weren't available before with the limited time that the teacher had.
We are serving millions, 13 million students as CA now.
It also unlocked and let us understand how...
the technology can have a real impact in the classroom.
And that's where it gets very, very exciting for us.
Having access to that real data, usage data is, I mean, unbelievable.
it sounds like you've gone from uh the change from a small company to a big company is one
big thing you had to get used to, but that brought with it sort of benefits in terms of
the access that you had and the ability to actually drive impact in this field, AI and
education.
I think we were super amazed by our colleagues in the US.
They are really passionate and scientific.
So they publish a report every year on the state of the student learning experience.
We were really amazed by the extensive studies that our psychometric team is doing in that
direction to analyze this data.
Yeah, so being acquired by a company that has that sort of data metrics mindset really
helped with bringing the two companies together and having them work in a great way
together.
Exactly.
Yeah, no, that was really, that's why at the beginning I said it was very easy to
synchronize our goals because they have a very scientific research grounded approach.
When you first started down the acquisition journey, were there particular signs or
particular things you noticed that have since come to point to the fact that you were
disaligned from the beginning?
Yeah, I think the enthusiasm was what thrown us to them and what made the acquisition
possible.
guess the excitement and the idea that they want to put children's experience first.
That was our main synchronization point.
The same was
Yeah, they were very welcoming, welcoming us in the team, always open to discussion.
Most of the speech tech team in Soapbox comes from a research and academia background.
So we are kind of used to, you know, sharing ideas, discussion, challenging opinions.
And people were quite open to that.
And people are so friendly and kind that we were...
Really surprised.
But I've been told it's quite unique.
I think sometimes you have to work together to discover a little bit about your alignments
and your synergies between two different companies.
So I'd love to delve a little bit more into how voice is being used in education because I
think we can all have an idea of how voice is being used.
But what are you seeing work in the classroom right now?
Voice in education, the traditional or the most immediate uh approach with voice, you ask
a child to read passages and then you assess how well they read and how the pronunciation
that the child uses is similar to the model that the AI model that was learned by data.
This is just
one of the ways where voice can be used, probably the low hanging fruit these days.
But one of the things that we are doing now that we are part of this bigger company is
understanding how data points, all the data points that you can extract from speech can be
used to inform a new metric or can inform a new way of
create instructions that are tailored to the student experience.
So instead of just providing a score in front of the child or the teacher, you can provide
them with a much wider uh range of options, like new exercises, new tests, or you can give
them suggestion to the teacher to help and support the child in a more
effective way.
So voice AI of speech recognition in the classroom, it will end up, I mean, in our vision
at least, it will end up to be one of the many data points uh sources that will inform the
student experience.
And last but not least, AI is not a teacher.
AI tools are not substituting a teacher.
AI is not built to be a teacher.
AI is a tool.
that creates data points, extract data points from speech, from writing, from exercises,
and all those data points that will inform a final assessment of student experience.
And that's where I think is the thing that gets us very excited.
Great.
So voice has got a lot of uses in the classroom, both in terms of sort of scoring how well
children are reading and evaluating that and also providing some personalisation and
feedback to teachers.
Exactly.
Our goal is mainly to transcribe as accurate as possible children's speech.
Of course, children's speech is a huge challenge because children don't behave like
adults.
They use different pronunciations, different grammar rules, maybe none at all sometimes.
So for example, language models and prediction of what comes next kind of fails with
children most of the time.
So it's more unpredictable what they're going to say next than with adults.
And their pronunciation, of course, needs to take into account of cultural background,
ethnicity and social background, all sorts of variables.
And the way we're trying to make our speech model robust is test, test, and again, test.
We are collecting a huge amount of data.
uh commission data or real data from classrooms and then once we identify there is a place
that can be improved then we focus on trying to find either more data or new techniques
that will help with that.
Our goal was to make the speech technology available for every single child and every type
of classroom.
Classrooms can be very noisy.
A lot of children can talk at the same time or again, their behavior is a little bit
unpredictable.
But by collecting data and making sure that our technology works in that scenario, we also
run a lot of tests on how our technology can be used in a classroom.
Yeah.
And I think we have a very scientific approach on that and we are doing great work.
And so far we've been surprised by the accuracy, even in quite noisy environment.
Is there a particular age range of children's speech that you're looking at?
Because I know that some of those things you mentioned about children speaking less
grammatically and pausing more and not forming their words as well.
And also that the frequency of children's voices is higher as well.
And some of these things are more pronounced when they're younger.
So do you find that your technology works best for a particular age range?
Because our focus is K12, from kindergarten up to grade 12, the younger the child, of
course, he will pose more challenges because of less grammatical rules they use or less
structure in their speech and they are less willing to talk as well.
They get tired very quickly or bored very quickly, so they don't follow the script very
easily.
So I wouldn't say the challenge with the technology, for the technology, is the actual
type of speech that allows to extract fewer data points than in older children when you're
the age of 10, 12, then they can read a huge paragraph.
So it will give you way more data points to inform a much better assessment.
Do you find that children enjoy sort of using voice technology in the classroom?
Do you get any indication of how it compares to other methods of learning and following
their progress?
We had quite good experience.
As I said, we ran a few user trials.
And most people, find it like a game.
So we've seen children really enjoying and getting excited because of the technology.
These days, I believe children are super used to use tablets, screens.
And that will become, for example, letter name reading.
and we had this child reading through the screens and trying to be as fast as possible,
maybe sometimes too fast.
And that was really a great experience that made our day.
Maybe other children, they'll need a little bit more time to get used to the screen, but
we are spending a lot of time into investigating which is the best methodology that works
for everyone.
And we're also very much aware that AI is just one tool.
It might not be the best tool for every child.
There might be a differentiation in that direction as well.
And one of opportunities of AI is to give uh the teacher's time back to them.
Voice technology is, it goes through phases, right?
Everybody gets excited about voice technology and then dies.
I've seen this several times over the past 20 years.
on the new hammer that comes on the market.
Exactly.
And I think we are at a time where people are again excited about voice technology with
some of the recent advances that are happening.
So this is a question I think everybody asks me or has to try and get to grips with is how
to keep up with all of the advancements that are coming your way if you work in this
field.
So what are your thoughts on how to keep up with the state of the art and what's happening
in the world of AI?
Yeah, I'm not going to lie.
This is one of the biggest challenges, as you said.
I guess the way we are uh keeping up with all the changes is through reading.
The team I work with, they are super passionate, not just data scientists, but they are
researchers at heart.
They are passionate about what they do and they read.
And also, we love to go to conferences.
We try to go to conferences as much as possible, being exposed.
what's new ah and maybe set aside a little bit of our time to investigate a new technology
or a new model or new approach to speech recognition.
These days we are not just limited to speech recognition, we have generative AI of course
as well that we are working with.
So every new style, every new chat GPT model that comes our way, we have to test it.
We have to see how that works.
I would say we have to save 10, 20 % of our time into investigating new technology.
Again, the choice that we made, I think, going to conferences and collaboration with
universities as well, if we can.
uh We have a couple of conversations going on.
with some universities in the US or in the UK or in Ireland as well, where we are trying
to make sure that we have uh two ways communication.
We can have interns coming our way and work with us and using their methodologies on our
data and also we can ask them to investigate some new technologies for us.
Are there any particular, I know there's been a huge change in AI in the past few years,
is there anything in particular you can point out and say this one breakthrough, this one
advancement has really helped us?
I mean, I guess pre-trained models changed everything in speech recognition, especially, I
think, the accuracy that some pre-trained models show because of the huge amount of data
that no even medium-sized company can afford.
I mean, it was a game changer.
Our approach in that direction was to focus on not just on accuracy,
but also production-ready tools because again, we are building products.
If we have the best model ever, ultimately we want to deploy it into the market.
So we also care about, know, inference being very robust, stable and production-ready.
Some tools that are maybe very popular in academia, maybe won't be so production-ready.
And the other thing is documentation.
Again, because most of these models needs to be deployed, the engineer side of the house
needs to have a strict guidelines to how to handle these models.
And that brings us to MLL engineers and always a difficulty is to find good ones, but
yeah.
m
Yeah, that is a real challenge, it?
Taking models from this prototype research-ready version through to a production-worthy
system.
So accuracy is not everything.
It's also how easy it to make it into a product.
So just to sort of wrap up our conversation, what perhaps are the lessons that you have to
share with people who want to move into this field, want to build AI technology,
particularly if they're maybe thinking about education, what are the top things that you
would share with people?
Well, if you have to focus on building tools for education, think data is key.
You can leverage, of course, a lot of pre-trained models, but you have to understand that
children's data is different from adult's data and pre-trained models are not ready for
children's speech.
So collecting data and testing on in-domain data, the type of use cases that you...
want to target, that's the key.
The other thing is, say, especially going through acquisition and other thing, the other
lesson I learned that every day is different.
And I might say that I probably learn even more in three years in the company than in my
years as a PhD student.
As a PhD student, you have one topic.
You need to go deep into that topic.
But when you are in a company, maybe you have to diversify much more.
and try to work on many different tasks.
So it sounds like having the right sort of data and making your models work for those
sorts of data is key for building products, but also then, you know, taking your skill set
and making it work in a company is also another lesson that people can learn from your
experience.
So thanks so much.
This has been really interesting.
Where can we go to find out more about you and Soapbox Labs and Curriculum Associates?
I guess the easiest way to connect with myself would be through LinkedIn.
And as for Soapbox Labs, we have a website.
So SoboxLabs.com is the best way to connect.
The same with Curriculum Associates.
Curriculum Associates.com is the website.
I shall put links to all of those sites in the show notes of the podcast so people can go
and find them and click through.
Well, thank you so much for your time.
was fascinating to hear about your journey to acquisition and building educational tools
with AI.
really, thanks very much for your time.
Thank you very much for having me.
That's it for today.
Thanks for listening.
I'm Catherine Brisson and I hope you'll join me again next time for Fireside AI.
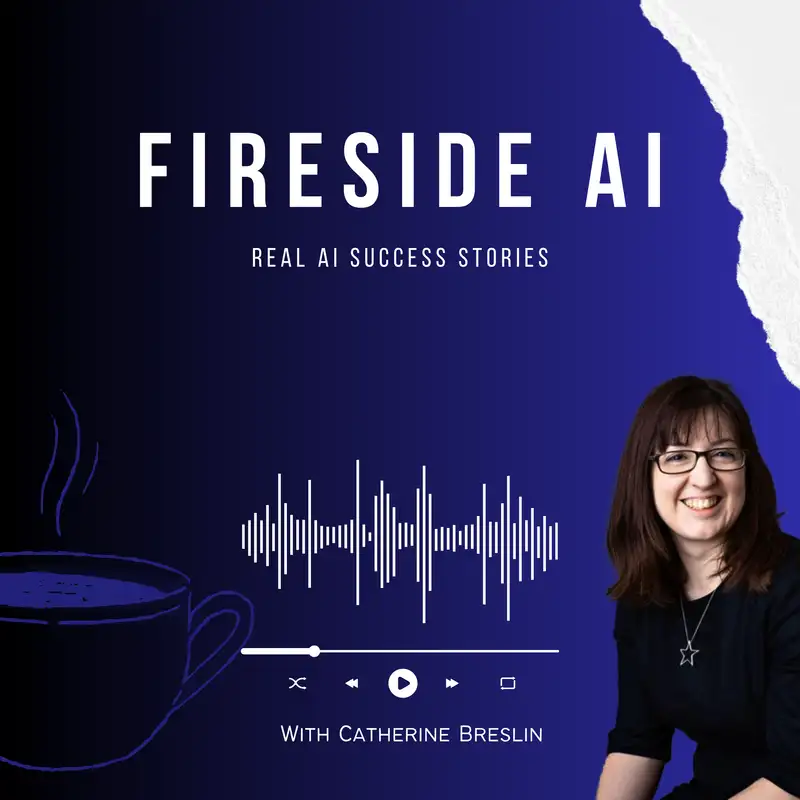