Empowering Data and AI teams with Chris Pedder from Obrizum
Hi, and welcome to Fireside AI. My name is Catherine Breslin, and I'm here to talk about how companies build AI technology. Today, I'm joined by Chris Pedder from Obrism. Chris, welcome to the show.
Speaker 2:Thanks for having me, Catherine. It's great to be here.
Speaker 1:Fantastic. It's great to have you here. For people who have not met you before, let's start with a brief introduction to you and Obrism, what it is that you do.
Speaker 2:Sounds great. So there's a bit of a giveaway in the background behind me. You can tell not everyone has a blackboard in their home office. So there's a bit of a hint of my past. So I'd like a lot of people in the machine learning world, I'm a lost physics researcher.
Speaker 2:I did lots of research into high energy physics in my time. And then about eight years ago, moved to Switzerland, tried to move into doing physics research here and discovered that I was too old and not fashionable enough and discovered another point, is this whole area of machine learning was kind of kicking off and people were really interested in using machine learning models to do all sorts. So I switched across into doing machine learning work and I landed up eight years on as chief data and AI officer at Abrism. Abrism are a learning and development company, so our focus and our mission is really on being the world leader in the measurement of human learning in digital environments. Sounds like a strange focus for a company which is really all about using machine learning and AI to improve learner experience.
Speaker 2:We actually landed on measurement because there's this really big problem in education in general that we don't really know what moves the needle in terms of educational outcomes. We discovered that in order to do a good job of guiding people through their learning journeys, we first needed to work out how to work out where they were. So we're really trying to build a map and a compass which guides people through learning material that they might need to learn as part of their job or for compliance courses or for any number of the different applications out there in the world. The focus on measurement has actually turned out to be really useful because, yeah, it's something that will guide us in how people learn. It's not a well known thing from psychology, so we have to start from those principles.
Speaker 1:Great. And I know physics is such introduction into machine learning and AI. A lot of people come up through that roots, and I think it stands people in in great stead. And over some I know the focus on education and being a relatively small organization too. I'm curious to maybe talk about some of the, like, the behaviors that you, as as chief data and AI officer, really feel is important to instill and foster in in your AI machine learning teams.
Speaker 2:It's this is a really good question. I think the key thing for me, especially for smaller organizations, is when you get to big scale, if you're a Google or an Amazon, you can really build specialist teams. So you can have a platform team which are there just to serve data to the machine learning teams, to provide feature stores, to really be there as the supporting lattice work and network to to guide data science and machine learning teams. When you're smaller, especially at the start up level, you really need people to be prepared to roll up their sleeves and do a wide variety of stuff. There are all sorts of memes circulating on LinkedIn.
Speaker 2:I'm sure you've seen them where people are talking about, well, I signed up for this data science job, but actually I'm doing 80% of my time doing cleaning data and a bit of building data pipelines. And one day I hope I can do some machine learning. The reason it's fun funny is because it's true to some degree. So it's really important that machine learning teams, especially early stage, are prepared to do stuff differently. When I first joined the BRISM, we had a data science team of three data scientists, and we had all of these amazing solutions which were hived off in their own chunk of our software real estate that weren't really accessible to other people.
Speaker 2:So the first thing I did, and thankfully my team were very forgiving of the fact that I tried to force software engineering on them. The first thing I did was come in and say, we need a way of actually making this available to the rest of the business. And so we built out all of the infrastructure ourselves in AWS to support the machine learning models. It's a really nice way of going about things as well because it gives you a sense of buy in as part of that team. So you really feel like you've done something when you deploy a new API endpoint and when you can test it and demo it.
Speaker 2:Whereas if you're constantly depending on other teams to get your work out there and into production, it can be a bit disappointing because you feel like you've done something great, And then you sit there for a month with your model weights from a Jupyter notebook somewhere trained on your own machine, and the engineering team aren't that thrilled about the fact that you're throwing stuff over the wall to them and hoping that they pick it up. So it it's been quite interesting to see the empowerment that you can develop.
Speaker 1:And do you think then that you look for specific skills on the people that you're hiring? Because being broad in machine learning, at least say, it can be great for that sense of ownership and being able to deliver things. But does that change the way you're looking at the skills that you're trying to hire onto your team and develop?
Speaker 2:I'm I realize I'm a bit strange in this, but I really strongly believe in that mantra of hire for potential, not for ability. So I'm much more excited by people who are excited by the challenge than I am about finding someone who's managed hundred nodes, Kubernetes clusters, and knows how to do all of that stuff. I think it's much more important for someone to be enthusiastic about discovering new stuff and wanting to develop and wanting to learn I'm probably guilty of hiring for people who have the same attitude as me. I like to learn and I like people in the team who want to learn with me. We can dig into stuff which maybe doesn't work and try and make it work together.
Speaker 2:So I I I'm probably guilty of being a little bit too hands on with a leader. I think it's nicer that way than being completely cold and hands off and saying, well, it's your problem. Let me figure that out.
Speaker 1:Yeah. On a small team, I guess everybody's looking to you to help point the way to to where they're going. And do you find then there are certain things you you hire great people with a lot of potential and enthusiasm, sort of instill that sense of ownership in them. Are there things that you do to encourage that?
Speaker 2:It's really, really important to give them the opportunity to have their own voice. Actually, I've been trying really hard with my team in Abrisom to step back and and allow them to to grow into their own roles and allow them to take ownership of things. So one of the team is very already a very competent engineer and very capable. And she asked me, what do I need to do to move forward in my career? Technically, you're great.
Speaker 2:How about we do something a bit different? So why don't you take charge of organizing seminars for the rest of the company so they can find out what we do and so we can guide them to really understand the details of what we're building and also tell the commercial teams and the sales teams, this is where we're strong and this is where we're weak so we can guide a bit the the business strategy of the company. And she's taken this like a a fish to water. So it's really it's great to see you can build confidence in people in a whole host of different ways. And it's it's really lovely to see it when it works.
Speaker 1:And working in a small company, I guess, gives you more of those opportunities to interact with a lot more different kind of roles and different Definitely.
Speaker 2:Yeah. Having worked in big enterprises, it's definitely harder to do that. And you don't really have the scope to go broad. So it's really nice to see, especially kind of a startup person by nature and by disposition. So I like the environment where I can give my team fun challenges to run after and be there to try and catch them when they fall.
Speaker 1:Great. And does that lead then to any specific thoughts that you have in mind about how you organize your teams to work effectively so that they own everything and that they're not, you know, throwing model weights over the wall and hoping for the best?
Speaker 2:Definitely. When I joined the BRISM, gosh, two and a half years ago, wow, that's gone quickly, we had a conversation between me and the other engineering leaders as to how we wanted to structure all of our engineering teams. And we settled on using team topologies as our guiding principles. Makes life very easy from the engineering and product sides. So you align your engineering teams to particular parts of the product real estate.
Speaker 2:And then you try and get around Conway's law, which is that you end up building a product which represents the way in which the teams are structured. You try and invert that and make it so that actually you structure your teams so that you build good products. But it did leave us with a bit of a puzzle on the data side. I ended up going away and putting a bag of frozen peas on my head and trying to think, well, how do we actually fit in? Because data teams are always a little bit they're always sort of square pegs in round holes, but I ended up coming up with the idea of, well, we have data engineering who are clearly a platform.
Speaker 2:They provide infrastructure for the rest of the organization. Our data engineering team provide data internally, but also to our customers. So we provide all sorts of dashboards and can see how people are doing in raw. Data science and machine learning is a bit more tricky. And this is how we came up with this idea of, well, we'll just we'll build our own real estate and we'll provide access to the engineering team.
Speaker 2:We decided we were kind of a complex subsystem team. We're too small to embed individual machine learning people in the stream teams, so we decided we'll we'll work as a kind of build structure. We will go into collaboration mode with different engineering teams when they need particular solutions. But ultimately, we don't want them to have to worry about what's going on under the hood and what particular weird AWS infrastructure we need to deploy machine learning models. That should be opaque to anyone using our services.
Speaker 2:So we'll just provide those services as a as a complex subsystem team. And that's how we've been working. I dream of the day when we can actually hire more people in to help us maintain all of that real estate. It's coming. I know it's coming.
Speaker 1:And I'm sure there are plenty of people who've read team topologies and some who maybe haven't. And I know it focuses on sort of four modes of team operations. So you've
Speaker 2:got
Speaker 1:your stream aligned team building the product features, platform team, which is you said your data engineering team are building internal tooling. They have a sort of consulting model and what you call the complicated subsystem team.
Speaker 2:Yes.
Speaker 1:And do you find I know it also has some mechanisms of interaction between different teams. So do you find those useful ways of thinking about the interaction? Definitely.
Speaker 2:So we decided that our primary mode is complicated subsystem teams. When you read the team topologies book, sort of feels a bit like a bucket where you put everything that doesn't fit into the other three, if I'm honest. For that reason, it also allows you more or less any interaction mode you want. We've decided to prioritize x as a service. So providing services that engineering teams can call because that way it allows the different teams to move at their own pace.
Speaker 2:And it means that their engineering teams are not so dependent on us, and we're also not so dependent on them. But we also like to do the the consulting model where you go into collaboration mode. If we're working on a particular streamline project, is gonna be long lived, then we'll go in embed with particular teams and make sure that we're start joining their stand ups and helping out day to day.
Speaker 1:So you're taking the bits that work for you at the time depending on on the sort of projects that you have on and
Speaker 2:Exactly. The
Speaker 1:the needs of your product. Yeah. And I think that's great because I think that dynamic of the data teams working with the engineering teams can be a bit of a difficult one to juggle if you have especially if they're different leaders.
Speaker 2:I've definitely seen this be a really stressful environment. In previous places I've worked, it's it's led to all sorts of political reactions. And in some cases, I've also seen leaders called into meetings with the CEO to try and hash out who's actually in charge. Luckily, in Brisbane, we all get along very well, and it's we're all basically trying to dance and not tread on each other's feet. So it all works quite nicely.
Speaker 1:Nice. So so looking at those sort of modes of interaction and ways of building teams and then giving teams the ownership of what they are are building and ownership of the machine learning infrastructure as well as well. Yeah. Well for you. And maybe let's talk a little bit about so the domain that you're in, education obviously has its own quirks and characteristics.
Speaker 1:How does that play into how you're using AI for your product?
Speaker 2:It's a fascinating place to learn about where AI is strong and weak. There's this fundamental problem that in most other places that you work with machine learning, you're trying to make your whole experience for your end users less full of friction. So you're actually trying to make things slicker. Spotify have a recommender system because they don't want to say as soon as your song ends, now you need to pick another one. So the idea is to reduce the user friction.
Speaker 2:But learning is an environment where, actually, struggle is really important. So I distinctly remember going skiing with a a friend of mine a couple of years ago, and his kids saying to me, the struggle is important. They you know, eight and 12 and in school, my god. I'm being taught all about education by an eight year old and a 12 year old. That's kinda cool.
Speaker 2:But so they really emphasized to me, it should be effortful. If you want to learn something and it's a valuable thing to learn, it's going to take some struggle to get there. That means that when we use machine learning and AI, it isn't necessarily to remove user friction. In fact, sometimes it's putting extra user friction. You need people to be in an area where you're challenging challenging them enough that they're rewiring the neurons in their brain, but not so much that they get annoyed and slam their laptop closed.
Speaker 2:So that means that it gives you a different perspective. It also means that it's really the thing that keeps me awake at night and the thing that I dread. And luckily, I'm in an environment where I don't actually have this as anything other than a stress stream is the idea that someone posts a screenshot of your learning environment telling obvious lies. We all know that large language models are still not great and they still hallucinate a lot of stuff. There were lots of companies that went all in on chat GPT early on in the development cycle and have not rode back from that.
Speaker 2:I would not want to be the chief data officer in those companies because it would keep me up at night, the idea that we're relying on something which is intrinsically unreliable to give important information to learners. So it's also made us think very carefully about how we do this in an ethical way.
Speaker 1:And of course, yeah, absolutely. You don't want your education tools to be giving you the wrong information. And I think a lot of I sometimes use chat GPT to find out stuff and sometimes it's things I know about and I I see those mistakes. And so how how then do you embed this sort of ethical thinking throughout your team and make sure that you are actually focused on, know, firstly, building technology that's not going to give students the wrong information. And secondly, that you're gonna evaluate that you're doing that and and make sure you're you're measuring.
Speaker 2:I mean, I have a great story of this as well. So from my physics days, I decided I would ask ChatGPT about the work that one of the professors that I worked with previously in Luxembourg, I just wanted to get a summary of what Thomas had done. And ChatGPT hallucinated a whole load of references, including one that included me as a co author, which did not exist. And that was my moment very early on in my job in the Prism. So ChatGPT came out three weeks into my my current role.
Speaker 2:I realized that that was it. This is a problem. We actually need some process around this, and we need to think about how we're gonna deploy machine learning solutions. Otherwise, it would be very easy to sleepwalk into a world where we use this badly and in a way that's gonna ultimately set fire to the company. So we set up amongst this three data scientist plus me an ethics procedure.
Speaker 2:So we came up with what we think is important and a way of scoring solutions that we come up with against a grid of this is acceptable risk, whereas this is totally unacceptable risk. We've been using that to guide our decision making ever since. So simple things like making sure that if you're going to include educational information in something that learners will see, it should be vetted by a human being. And there shouldn't be the thing that we're always asked about is, can you build a bulk accept? Nope.
Speaker 2:This is vitally important that people actually go through and they read what's there and they're engaged with it, and we don't allow them to do that for too long. Otherwise, they're just going to get bored and scroll to the bottom and click yes. So we also actually have to build friction into the experience of managing learning spaces in order that we can use things like generative AI.
Speaker 1:So you have a ethical framework that you put in early on that you you stick to, and you have human in the loop, like, at various points to make sure that your LLMs are not generating anything that that could be contentious or incorrect.
Speaker 2:Absolutely. Yeah, indeed. And there's one other place. So the one place where we show things to learners where there's not human review is we do have a rag system. So we use retrieval augmented generation to find answers to learners' questions in a space.
Speaker 2:We spent a lot of time making sure that our prompt is as good as it can be. And we've also tried to use models. We use Anthropics tools to produce our summaries. We've used models which are good for their ethical background and we're running it over quite small spaces where we can do classical search first to find the right results and then summarise over those. It's easy to test that we're we're doing a good job there.
Speaker 2:But there's still that worry that you have of you have to have the right LLM ops approach and you have to allow people to flag stuff as being potentially potentially iffy so you can go back and check your prompts too. You're never done.
Speaker 1:No, no, never done. There's always something new gonna come up, right? So this has been fascinating to hear about, you know, the importance of having teams own own their code and and organized effectively to be able to build good technology and also have that ethical, like, awareness in their minds from the from the early days. So if you were to share, you know, your biggest lessons over your time here, what would you what would you say? What would you what wisdom would you give to people?
Speaker 2:I think for me, it's been it's been a fascinating experience. So I inherited a team of three three data scientists and machine learning people who were already hired before I joined. To be honest, I haven't hired anymore. So I was one of the things that I think is really important to evaluate as a a leader is how lucky you've been. I've been phenomenally lucky with the team that I inherited, so I I really landed up with a great team to start with.
Speaker 2:With that in mind, if you have a great team, don't be too frightened about giving them challenges. So my team have really taken taken on the challenge of doing software engineering and doing stuff which is not fiddling in Jupyter Notebooks and then throwing weights over the wall. They've taken to this incredibly well, given that it's something that none of them had really been exposed to previously. They're now really enthusiastic about this. And there are times when they shout at me for making poor requests that break the unit tests and that kind of thing.
Speaker 2:So life comes at you fast, especially if you have a good team.
Speaker 1:Good to have a team who will push back if you
Speaker 2:do Absolutely. That's completely honest.
Speaker 1:Great. Well, thanks so much. This has been great. Where can we find out more about you online?
Speaker 2:So you can find out about the company at brism.com. We're we also do events from time to time in in The UK and also in The US now. And you can find me on LinkedIn. So especially if you're interested in getting into machine learning, I like to do my public service by helping out people who are new to the field. So don't be frightened.
Speaker 2:Look for someone who looks like a mountain bum wearing an orange fleece on LinkedIn. Send me a message. I'm happy to help if you need some guidance as to how to get into the field.
Speaker 1:And then I will put links to both of those in the show notes so that listeners can can find out where to where to find you. Fantastic. Well, thank you very much for joining me today.
Speaker 2:Thank you so much, Catherine.
Speaker 1:That's it for today. Thanks for listening. I'm Catherine Brisson, and I hope you'll join me again next time for Fireside AI.
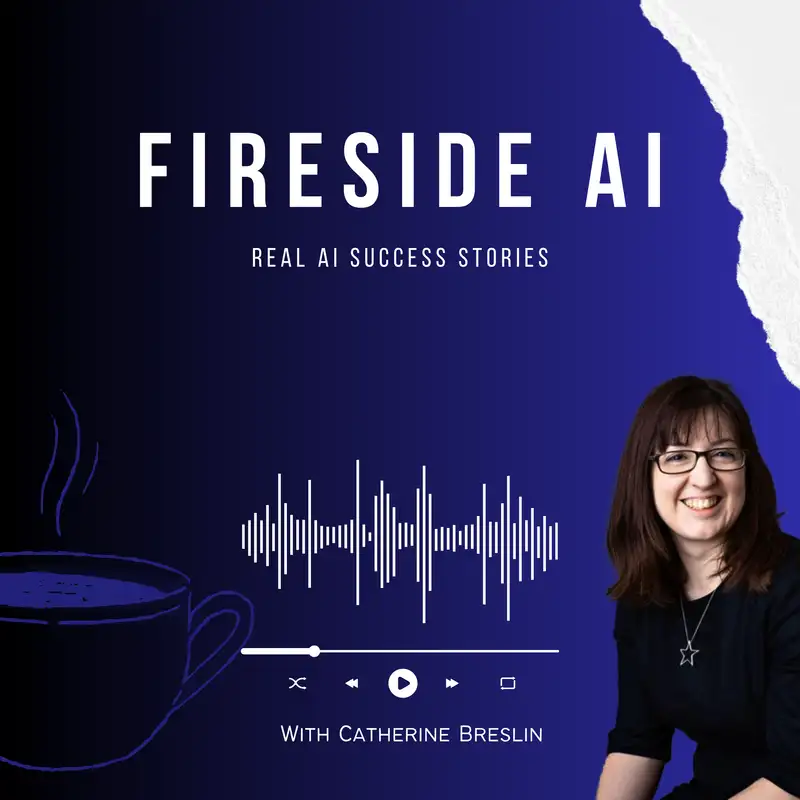